EDI, a fighter jet based on the F/A-37 Talon, is a fictional lethal autonomous weapon (LAW) designed to carry out airborne attacks on enemies and come back home in time for dinner without any human intervention. Why?
A machine pilot would not suffer from the physical limitations a human pilot would have to endure, while also being able to calculate alternatives faster and more accurately. Moreover, it would not have a human ego.
Sounds safe, right? More on this later! Let us first take a look at what it takes to build weapons that have the license to kill.
Fundamentals: What sets autonomous weapons apart
The biggest impact that LAWs have is that these let us eliminate us from the battlefield, that is, they remove the human operator sitting behind the gun. This is fine as long as the machines do not take it literally and go about eliminating all humans.
Unfortunately, machines tend to take things literally, more often than not (like when you run a program on a computer).
It means that LAWs not only need to be able to have the sensor capability to select and identify targets, but understand friends from foes, and also decide which type of tool to use in order to take down a particular kind of target with minimal collateral damage.
Finally, it would have to be able to activate the selected tool (guns, rockets, lasers, electronic jamming, flares, and so on) and deliver it with reasonable accuracy.
Now, the first and last parts of this flow, which are the sensors and actuators side of it, have been taken care of in many of our other articles as well as by the Internet of Things (IoT) as a concept.
But why do we need to have artificial intelligence (AI) strong enough to take humans out of the loop?
Looking into a Black Box
The capability to learn and adapt is a very serious business and can go either way. If you remember, the day after Microsoft introduced an innocent female AI chat robot to Twitter, it transformed itself into an evil Hitler-loving character that spewed hate and swore at Twitterati who tried to speak to it. Why?
Neural networks like the one in the fictional EDI do not perform rule-based calculations.
Instead, these learn by exposure to large data sets. These data sets could be easily powered by the Big Data generated from the IoT.
As a result, the internal structure of the network that generates output can be opaque to designers—a black box. Even more unsettling, for reasons that may not be entirely clear to AI researchers, the neural network sometimes can yield odd, counterintuitive results like the Twitter bot we just talked about.
“A study of visual classification AIs using neural networks found that while AIs were able to generally identify objects as well as humans, in some cases, AIs made confident identifications of objects that were not only incorrect but that looked vastly different from the purported object to human eyes.
The AIs interpreted images, which to the human eye looked like static or abstract wavy lines, as animals or other objects, and asserted greater than 99.6 percent confidence in their estimation,” explains Paul Scharre, senior fellow and director of 20YY Future of Warfare Initiative, in Autonomous Weapons and Operational Risk.
Deep neural networks and Narrow AI
Existing technology for Tesla’s semi-autonomous driving cars uses deep neural networks (DNNs). When the human brain tries to recognize objects in images, it does not see pixels; instead, it sees edges on them. A DNN tries to recreate how a human brain functions by programming it to only recognize edges.
This machine or program uses code to look at objects and do some unsupervised learning of its own—known as machine learning. When enough time has passed, the machine learns to distinguish between whatever the DNN was told to look out for. The intelligence of the DNN depends on its processing power and the time spent learning.
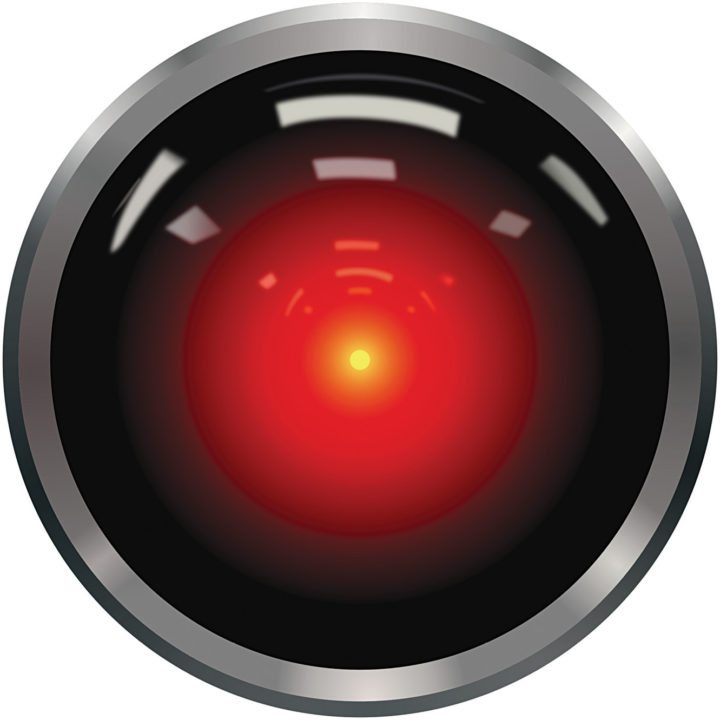
Companies like Tesla are developing Narrow AI, which is a variant that stands for non-sentient computer intelligence that is focused on a narrow task that prevents it from doing something like trying to take over the world. Apple’s Siri is apparently a good example of Narrow AI.
Specialized hardware to run neural networks
Neural networks used for image analysis are typically run on graphics processing units that specialize in image processing. But much more can be done to make circuits that run neural networks efficiently.
Joel Emer, Massachusetts Institute of Technology (MIT) computer science professor and senior distinguished research scientist at NVIDIA has developed Eyeriss, a custom chip designed to run a state-of-the-art convolutional neural network.
In February 2016, IEEE International Solid-State Circuits Conference in San Francisco, USA, teams from MIT, Nvidia, and Korea Advanced Institute of Science and Technology (KAIST) showed prototypes of chips designed to run artificial neural networks. The KAIST design minimizes data movement by bringing memory and processing closer together.
It saves energy by both limiting data movement and lightening the computational load. Kim’s group observed that 99 percent of the numbers used in a key calculation require only eight bits, so they were able to limit the resources devoted to it.
Nvidia also announced a new chip called Tesla P100, which is designed for a technique called deep learning used by Google software AlphaGo. They also unveiled a special computer for researchers involved in deep learning. It comes with eight P100 chips, memory, and storage.
Models of this computer, known as DGX-1, will be sold for US$ 129,000. It is being given to leading academic research groups, including ones at the University of California, Berkeley, Stanford, New York University, and MIT—all in the USA.
Solving the problem of skewed measurements
The two eyes humans possess help with depth perception. This is helpful in many cases.
Similarly, when we talk about machines, we usually have single-aperture systems, which raises the question of skewed measurements. Arvind Lakshmikumar, chief executive officer, of Tonbo Imaging, says, “Aperture systems have their own set of limitations that can be overcome with multiple aperture systems.”
Efficient imaging systems employ sensors to the optimum. Their signals have to be safely transferred to the computing unit. Post imaging, signal transmission is another part to be looked at. “Intelligence guys often tap signals,” says Parag Naik, co-founder and chief executive officer, Saankhya Labs.
Hence, signal security becomes an issue while transmitting the signals as well. Some security measures can be taken by making suitable changes in the system. However, even with the best of systems, “chances are still pretty high for collateral damage,” says Lakshmikumar.
How does somebody go about banning weapons?
The open letter signed by Elon Musk, Stephen Hawking, and Steve Wozniak, among others, made big news last year. It warned the world about the dangers of weaponized robots and a military AI arms race currently taking place between the world’s military powers.
The section on autonomous weapons reads, “If it is permissible or legal to use lethal autonomous weapons, how should these weapons be integrated into the existing command and control structure so that responsibility and liability remain associated with specific human actors?”
The essence behind calling for a ban on these weapons now becomes the scapegoat for any miscalculation that the machine might do.
International Committee of the Red Cross (ICRC) details some views on humanitarian laws and robots, “No matter how sophisticated weapons become, it is essential that these are in line with the rules of war.
International humanitarian law is all about making choices that preserve a minimum of human dignity in times of war and make sure that living together again is possible once the last bullet has been shot.”
Even the United Nations meeting in Geneva earlier this year saw delegates from over 90 countries discussing the future of autonomous weapons. IEEE Spectrum states that experts believe autonomous weapons are potentially weapons of mass destruction.
The United States’ Directive 3000.09 prevents the use of fully-autonomous weapons but allows their development.
What could be done?
“Weapons have been banned before, but for autonomous weapons the control is important,” says Dr Peter Asaro, co-founder and vice chair, of the International Committee for Robot Arms Control. On the argument of stopping progress in the field, he explains, “Banning biological and chemical weapons did not stop progress in the fields. We just stopped weaponizing these.”
Some argue that these systems would be unable to adhere to the current laws of war. War is brutal, and trying to create guidelines in war does not work.
Dr Asaro believes, “It is great to think that technology can do better but, at the moment, we do not have such efficient systems.” So should the priority not be to stop war and all weapons instead of making a fuss about autonomous weapons?
Naik recalls a very interesting quote by George Bernard Shaw, “A native American elder once described his own inner struggles in this manner: Inside of me there are two dogs. One of the dogs is mean and evil. The other dog is good. The mean dog fights the good dog all the time. When asked which dog wins, he reflected for a moment and replied: The one I feed the most.”
For reading other defence articles: click here
Dilin Anand is a senior assistant editor at EFY. He has a B.Tech in ECE from the University of Calicut, and an MBA from Christ University, Bengaluru
Saurabh Durgapal is working as a technology journalist at EFY